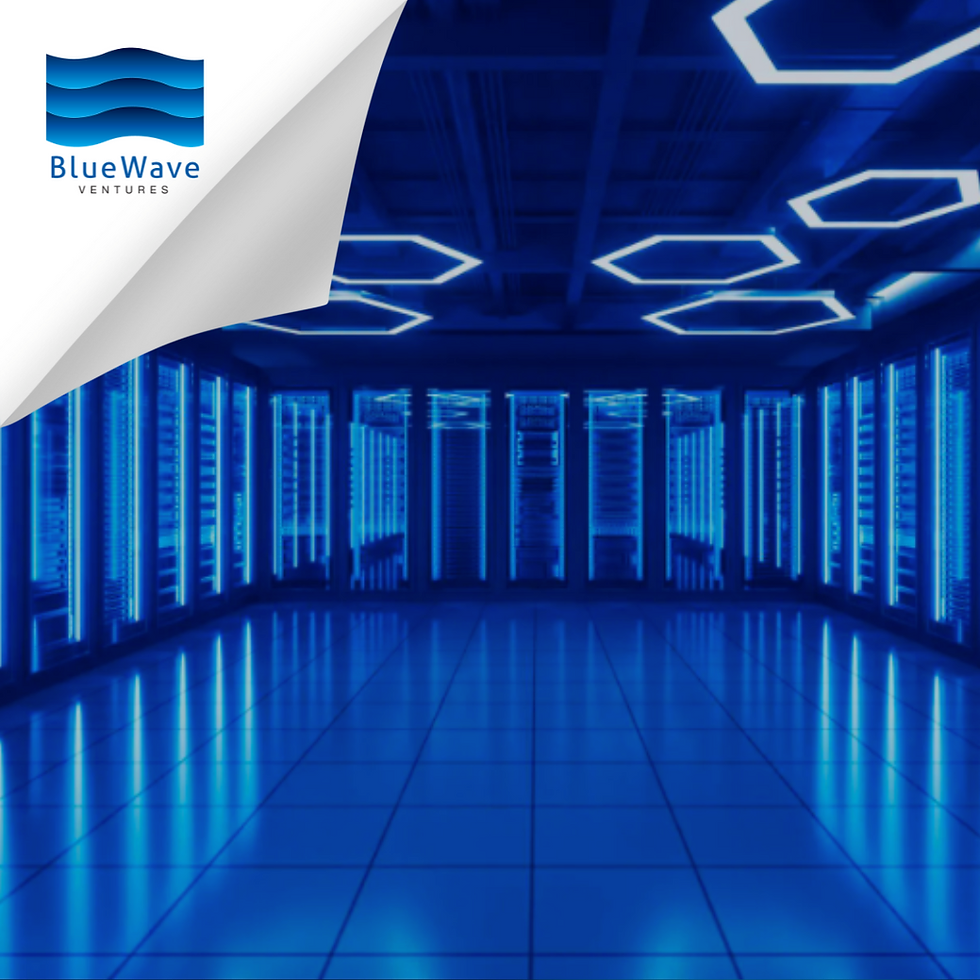
In Part 1 of this series, we looked at how app entrepreneurs can best leverage AI to create more software revenue, improve customer experiences, and build products in an AI-native way. Diving deeper, as a recent report from Bain Capital Partners (BCV) reveals, experts see how artificial intelligence applications also show promise in building the new infrastructure stack for restructuring data, agent-driven automation, and evaluation.
“Like code, models are complicated and costly to run,” write the authors. “Infrastructure tools not only address these issues, but also make models more powerful by chaining them together and improving model accuracy. This, in turn, enables AI to address more complex use cases.”
QUICK TAKES
Analysts expect the data center physical infrastructure (DCPI) market will grow at a 10% compound annual growth rate between 2022 and 2027, according to SDx Central
By 2025, there will be 55.7 billion connected devices, generating nearly 80 billion zettabytes of data, estimates the International Data Corporation
Data center infrastructure and operating costs are projected to increase to more than $76 billion by 2028, forecasts Tirias Research
While middleware connecting models to vector databases has received much attention recently, BCV points out that three equally promising areas deserve attention when it comes to developing and investing in AI: 1) unstructured data management; 2) agent driven automation; and 3) evaluation.
Unstructured data management
We all want better workflows, and we trust today’s sophisticated data capturing technology to deliver efficiencies. However, as the BCV report reveals, the most valuable data becomes slippery in real time, thanks to flooded inboxes and storms of Slack messages; technology is working so hard to log everything and record events, it misses what’s truly meaningful. Removing such structures with AI applications show potential to dramatically change how data can improve workflows.
“What if we could harness that data and use it downstream?” write the authors. "The result would be transformative for both enterprise and consumer applications. The topology of data would differ depending on the use case, from CAD file ingestion for structural engineers to fuzzy satellite images for naval intelligence, to PDFs for investment banks.”
Agent-driven automation
Earlier this year, ChatGPT hit 100 million users, making it the fastest-growing application in history. But such large-language models are limited by the fact they can perform only one task at a time, opening the door for generalized AI frameworks such as BabyAGI and AutoGPT to enter more workflows. What is stopping them is the bad reputation agent-driven automation has received from embarrassing errors such as recursive hallucination and memory failures. BCV authors note this can be overcome: “At the risk of egregious anthropomorphism, we believe future agent frameworks will behave as present humans do: with collaboration, cost, memory, and utility in mind.” Implementing multiple agents with distinct goals or functions and an “orchestration layer” to assign each agent a task can achieve this—a promising evolution.
Evaluation
Think of these applications as the Olympic judges of AI: they determine which LLM-based apps do the best of chaining open-source and hosted models. New technology is required for both model selection and model experimentation, explains the BCV report. “As open-source models proliferate and large hosted models compete on ergonomics, cost, vertical focus and degrees of enterprise suitability, a product is needed to evaluate models in real time for the task at hand and automatically choose the right one,” write the authors of model selection. “Similar to the recent growth we’ve seen in the cloud operations management category is emerging to help enterprises choose the right model automatically.”
An additional need is for applications that can assess the quality of AI responses to LLM-based features’ end users through metadata such as response time, latency, and validity. These AI apps should also be able to measure conversion and engagement, among other typical product metrics, adds the BCV report. Look for a new breed of A/B testing and feature-flagging paradigm in companies positioning for model experimentation.
Up next: Part 3 of the Best Bests For Artificial Intelligence: A Look Ahead
댓글